Towards an increasingly biased view on Arctic change
- Efrén López-Blanco,
- Elmer Topp-Jørgensen,
- Torben R. Christensen,
- Morten Rasch,
- Henrik Skov,
- Marie F. Arndal,
- M. Syndonia Bret-Harte,
- Terry V. Callaghan &
- Niels M. Schmidt
Nature Climate Change volume 14, pages 152–155 (2024)Cite this article
- 9416 Accesses
- 1 Citations
- 658 Altmetric
- Metrics details
Abstract
The Russian invasion of Ukraine hampers the ability to adequately describe conditions across the Arctic, thus biasing the view on Arctic change. Here we benchmark the pan-Arctic representativeness of the largest high-latitude research station network, INTERACT, with or without Russian stations. Excluding Russian stations lowers representativeness markedly, with some biases being of the same magnitude as the expected shifts caused by climate change by the end of the century.Main
As a result of the Russian attack on Ukraine, the Western world has excluded Russia from international fora. This geopolitical conflict severely challenges transnational collaboration on global issues. This is particularly evident when it comes to the Arctic. Russia is geographically the largest Arctic nation and is, hence, also one of eight nations within the Arctic Council, an intergovernmental forum for coordinated activities across the Arctic countries (https://arctic-council.org/). However, following the invasion of Ukraine, the work of the Arctic Council was first put on hold, and as currently resumed, it is only in part and without Russia.
The Arctic is rapidly changing1,2, and many of the ongoing changes may have global consequences3. While many of the key indicators of Arctic climate change (for example, refs. 4,5) and climate-induced responses (for example, refs. 6,7) can be estimated remotely, much of the understanding of Arctic change is based on in situ data measured on the ground at research stations. As ground-based observations that form the basis for assessments of the region’s state will now come mainly from the non-Russian parts of the Arctic, the overall ability to monitor the status and trajectory of the Arctic biome may be severely limited over the foreseeable future. The question is to what extent this challenge may bias the overall view on Arctic change. However, to better understand this challenge, there needs to be acknowledgement that the current view on Arctic change might already be biased8,9. Logistical constraints and limited long-term funding for conducting research and monitoring in vast and remote areas10 have led to the establishment of only relatively few research stations scattered across the Arctic without an optimal statistically determined sampling regime8,11. Most ground-based data collection and the resultant scientific publications are therefore spatially clumped8,9,12, and may thus not be representative of the Arctic region as a whole. Siberia and the Canadian high Arctic appear particularly under-represented8,9.
In this Brief Communication, we assess potential additional biases in the view on current and projected terrestrial Arctic change amid the current geopolitical conflict. To achieve this, we quantify how well Arctic research stations, with or without Russian stations included, represent ecosystem conditions at the pan-Arctic scale. We use a suite of eight state-of-the-art Earth system models (ESMs) from the Coupled Model Intercomparison Project Phase 6 (CMIP6)13, included in the Intergovernmental Panel on Climate Change (IPCC) Sixth Assessment Report14, at their native spatial resolutions (Extended Data Table 1). We focus specifically on eight essential abiotic and biotic variables describing key conditions in high-latitude terrestrial ecosystems2: annual mean air temperature, total precipitation, snow depth, soil moisture, vegetation biomass, soil carbon, net primary productivity and heterotrophic respiration. These essential ecosystem variables serve as benchmarks for environmental conditions found across the circumpolar region and at Arctic research stations located above 59° N, as represented by the pan-Arctic infrastructure network International Network for Terrestrial Research and Monitoring in the Arctic (INTERACT, https://eu-interact.org/)15.
Acknowledging that the INTERACT network may not be fully representative of the Arctic as a whole9, we first quantify any bias of the network in representing the contemporary spatial variability of key abiotic and biotic ecosystem conditions across the pan-Arctic region. We then ask whether the exclusion of Russia from INTERACT accentuates any potential bias. To quantify the discrepancies between the pan-Arctic domain and INTERACT research stations with or without those in Russia, we calculated two metrics. First, we calculated the maximum differences between the cumulative distribution functions (the D values from Kolmogorov–Smirnov (K–S) tests) of the pan-Arctic domain and INTERACT stations with or without Russian stations across the eight CMIP6 ESMs for each of the eight ecosystem variables (Fig. 1a). Significant D values (P < 0.05) were regarded as lack of representativeness between the INTERACT network with or without Russia and the pan-Arctic region. As a yardstick of magnitude, we compared these D values with those derived from the projected shifts in ecosystem conditions between the years 2016–2020 and 2096–2100 using the Shared Socioeconomic Pathway (SSP) 5-85 scenario. Second, to visualize the possible biases we also extracted the first (25%), second (median) and third (75%) quartile (Q1–Q3) values of the distribution functions for each ESM and ecosystem variable from the INTERACT research stations with or without Russian stations and compared those with the conditions across the entire pan-Arctic region (Fig. 1b). We do acknowledge that ecosystem models are associated with uncertainties (Methods), and are as such not an absolute descriptor of environmental variation. Still, ecosystem models are the best tool we have for inferring large-scale patterns in contemporary ecosystem conditions in a consistent manner and for projecting into the future.
Fig. 1: Shifts in representativeness.
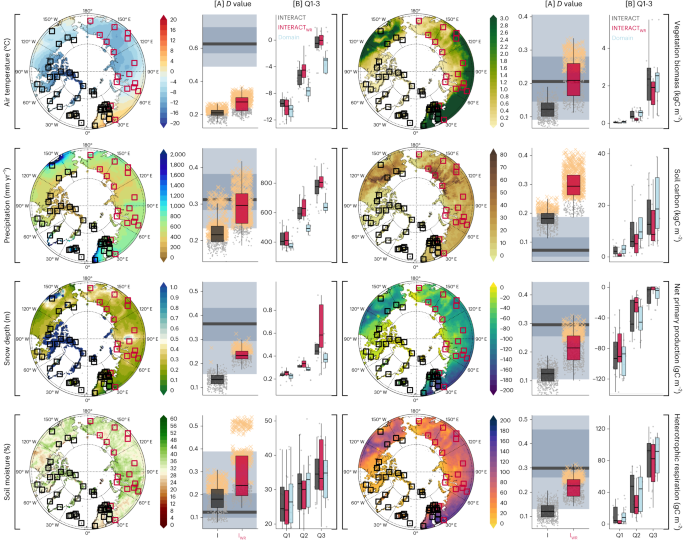
The effects of excluding Russian research stations (red boxes on the maps) from the INTERACT network with respect to eight ecosystem variables (air temperature, total precipitation, snow depth, soil moisture, vegetation biomass, soil carbon, net primary productivity and heterotrophic respiration). Maps visualize contemporary conditions above 59° N. For each variable, the potential biases of INTERACT with respect to the conditions in the pan-Arctic domain are depicted by two sets of box plots: [A] and . [A] shows the maximum deviation (D values) between two cumulative distribution functions (INTERACT with (I) or without (IWR) Russian stations) versus the contemporary pan-Arctic domain. The maximum deviation between the contemporary versus end-of-the-century pan-Arctic domain is shown by the horizontal grey bars, with the lighter and darker colours representing the median and the 25–75% and 2.5–97.5% confidence intervals, respectively. displays the quartiles 1 to 3 values for the ecosystem contemporary conditions of INTERACT with (black) and without (red) Russian stations as well as across the pan-Arctic domain (blue). Note that, for D values, both the eight ESMs and the resampling from the domain contribute to the variation, while variation for quartiles 1–3 is attributable to only the ESMs. All box plots show the median and interquartile range (IQR), with the upper and lower whiskers extending to the largest value ≤1.5 × IQR from the 75th percentile and the smallest values ≤1.5 × IQR from the 25th percentile, respectively. Outliers have been omitted to increase readability but are presented in Extended Data Fig. 1.
Source data
Full size image
Our results suggest that, even with all Russian stations included, the INTERACT network is consistently biased for some ecosystem variables and is thus not fully representative of the ecosystem conditions across the pan-Arctic domain (Fig. 1). The INTERACT stations are generally located in the slightly warmer and wetter parts of the Arctic in areas with generally deeper snowpacks. INTERACT stations are also located in areas with lower vegetation biomass and soil carbon than the Arctic region as a whole. This pattern is the same across the three quartiles examined (Fig. 1b), suggesting that the lack of representativeness for these key ecosystem variables is consistent across the parameter space. Hence, the knowledge based on ground-collected science may be biased, even when based on data from all Arctic INTERACT research stations. This corroborates the findings of previous studies8,9. Yet, local-scale spatial (subgrid) variability in ecosystem conditions around many research stations means that the environmental span covered by each INTERACT research station is broader than depicted by our large-scale analyses here (see, for example, ref. 16). The representativeness bias is thus probably different from what we have estimated here, but it is not possible to say whether subgrid variation generally contributes to lower or higher bias. On the other hand, as current ecosystem monitoring conducted locally at INTERACT stations is not fully coordinated nor standardized, the representativeness of the network for the pan-Arctic region may be even lower for some variables. It is only when research stations across the pan-Arctic region measure the same variables in a consistent manner across sites that we can achieve a more comprehensive and less biased understanding on Arctic change. Our measure of representativeness is thus rather a measure of potential representativeness.
Making matters more challenging, the exclusion of the Russian stations from the network (17 out of 60) resulted in a marked further loss of representativeness across almost all ecosystem variables, compared to modelled variables for the pan-Arctic region as a whole. For example, about half of the INTERACT stations located in the boreal zone are lost with the exclusion of Russia (Fig. 2), and with that, Siberia’s extensive taiga forest is no longer represented in the network. This results in additional biases, particularly with respect to vegetation biomass, with a concomitant increased bias in net primary productivity and heterotrophic respiration (Fig. 1a and Extended Data Table 2). Being a region characterized by rapid climate change17, the loss of Siberian research stations may be particularly detrimental for the ability to track global implications of thawing permafrost18, shifts in biodiversity, including shrubification19 and carbon dynamics20. Notably, for some variables (for example, precipitation and vegetation biomass) the offset increase was of a similar magnitude as the shifts inflicted by almost 80 years of projected climate change (Fig. 1a).
Fig. 2: Loss of ecoregion representation.
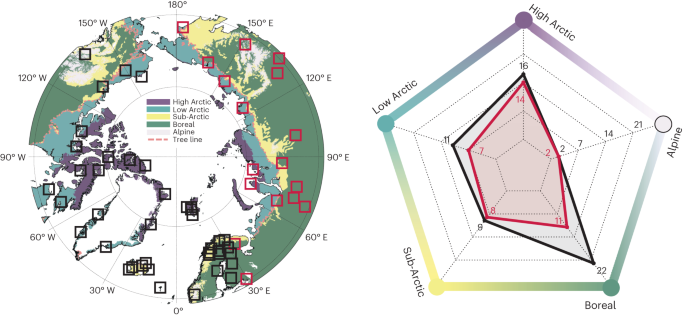
The impact of excluding Russian research stations from the INTERACT network on the count of research stations across the range of high-latitude ecoregions covered by the network. The INTERACT research stations are represented in the map by squares, and the red squares indicate the positions of the Russian stations. The radar plot to the right illustrates the number of stations within the various ecoregions, with the black polygon depicting all INTERACT stations and red polygon depicting the non-Russian stations only.
Source data
Full size image
Because of the geopolitical consequences of the Russian attack on Ukraine, the ability to both track and further project the development of the Arctic biome following climate-induced ecosystem change has deteriorated. And with that, the ability to initiate well-informed management and conservation initiatives that would help mitigate some of the negative consequences and risks exposed by climate change is greatly reduced. Understanding the gaps and biases is a prerequisite to, at least to some extent, consider and address them, and thereby improve the ability to make credible predictions despite imperfect coverage. Still, to be able to track the changing Arctic properly, the international community should, however, continue to strive for establishing and improving a research infrastructure and standardized monitoring programmes representative of the entire Arctic. This system should also promote open-access data sharing to increase accessibility and coherency. Sadly, until that is implemented, the ability to support and advise local and global communities will decrease further due to the loss of Russian stations representing half of the Arctic’s landmass.
Methods
Research stations in the Arctic
With 94 research stations in total, of which 21 are located in Russia, INTERACT (https://eu-interact.org/) is the most extensive network of research stations in the Northern Hemisphere. The INTERACT network aims to build capacity for documenting, understanding, predicting and responding to environmental changes achieved through the close integration of research and monitoring. The INTERACT stations cover a wide selection of climatic (high/low Arctic, sub-Arctic, boreal and alpine) and permafrost (continuous, discontinuous and sporadic) zones. To represent the network in the Arctic properly, we identified 60 grid cells containing the location of INTERACT stations above 59° N, excluding the Greenland Ice Sheet and INTERACT sites located in Svalbard sharing the same coordinates. Seventeen of these stations are located in Russia. The coordinates for the INTERACT stations have been obtained from the INTERACT Station Catalogue 2020 (available at https://eu-interact.org/).Spatial variability in ecosystem variables
We characterized the spatial variability of key abiotic and biotic ecosystem variables across the pan-Arctic domain using extracts from eight different ESMs (Extended Data Table 1) within the CMIP6 projections included in the IPCC Sixth Assessment Report14. Although today more ESMs are available, the ESMs included here were selected because they (1) include all ecosystem variables of interest (see below) and (2) are a diverse sample of most of the CMIP6 models as a function of effective climate sensitivity21. The CMIP6 datasets were downloaded from the open-source data repositories22,23. The model variant used for the eight ESMs was r1i1p1f1 (r, realization/ensemble member; i, initialization method; p, physics; f, forcing) to allow for appropriate comparability.We assessed the spatial variability in eight key ecosystem variables: air temperature (°C), total precipitation (mm per year), snow depth (m), soil moisture (%), vegetation biomass (kgC m−2), soil carbon (kgC m−2), net primary productivity (gC m−2) and heterotrophic respiration (gC m−2). These variables not only characterize the spatial variability in ecosystem conditions but are also known to be undergoing rapid changes across the pan-Arctic region1. The choice of variables was motivated by the key most recent trends and impacts from Arctic climate change reported by the Arctic Climate Change Update 2021: Key Trends and Impacts report1. For instance, air temperature is an excellent indicator that locally aggregates surface and atmospheric (vertical and horizontal) energy budgets. The temperatures in the Arctic have warmed three1 to four5 times that of the globe, increasing by ~3 °C during the 1971–2019 period according to EU Copernicus ERA5 monthly dataset. The total precipitation, together with air temperatures, are drivers of change for multiple ecosystem components. Precipitation in the Arctic is increasing nearly 10% in the same period and is driven by a 25% rainfall increase over-compensating for a loss of snow cover1. The Arctic system is typically covered by snow in the winter months, making the shoulder seasons (spring and autumn) especially sensitive to changes due to warming. The snow cover extent between May and June has decreased by 21% over the 1971–2019 period1; this is a percent loss rate greater than the loss of sea ice in September. Both rainfall and snow dynamics are among the key factors driving soil moisture availability that, at the same time, have important implications over plant phenology and productivity24. The tundra greenness has increased by 10% between 1982 and 2019 despite some regions exhibiting browning1. Greener tundra can increase the accumulated carbon storage and leaf area index further enhancing the photosynthetic capacity and stimulating higher gross carbon fluxes25 but also have important implications for land surface energy budget as does the reduction in spring snow cover26. Finally, the terrestrial C pool in the Arctic accounts for approximately 50% of the global soil organic C pool27—changes in soil temperature and permafrost dynamics can have strong implications on atmospheric release of greenhouse gasses and feedback to the global climate28. For each ecosystem variable and each ESM, we collated and processed monthly aggregated gridded information across the pan-Arctic domain. To describe the contemporary ecosystem conditions, we used the means of the years 2016–2020. To allow for comparison of spatial versus temporal changes (see below), we also estimated the spatial variability in the eight ecosystem parameters by the end of the twenty-first century (2096–2100) for each ESM. We used the SSP greenhouse gas emission scenario 5-85, equivalent to the former Representative Concentration Pathway 8.5 in the IPCC Fifth Assessment Report. We focused on this business-as-usual scenario as it has been recently found that we are very close to the upper part of, if not exceeding, the most drastic projection at least until the middle of the twenty-first century5.
From the monthly aggregated global CMIP6 ESM products, we then cropped out latitudes below 59° N and excluded the fractional Greenland Ice Sheet cover29. The spatial resolution of the individual ESMs was retained.
Data analysis
To assess the representativeness of the INTERACT stations of the entire pan-Arctic region, we calculated the density distribution for each individual abiotic and biotic ecosystem variable. As contemporary and future conditions, we used the mean across the years 2016–2020 and 2096–2100, respectively. First, we estimated the density distributions (Extended Data Fig. 2) for INTERACT with all stations included, and then with all Russian stations excluded. To describe the baseline conditions across the pan-Arctic domain, we randomly sampled the same number of grid cells from all ESMs, regardless of their native spatial resolution, equal to the smallest population size among all models (that is, the CanESM5 with 496 datapoints, excluding pixels containing ocean and the Greenland Ice Sheet; Extended Data Table 1). To minimize potential artefacts emerging from the arbitrary sample size choice, we retrieved 100 replicates of the random sample populations of 496 datapoints per ESM and variable. A simple sensitivity analysis assessing the impact of the number of samples and the number of replicates on the K–S statistics can be found in Extended Data Fig. 3.To describe any bias between ecosystem conditions between INTERACT with and without Russian stations and the pan-Arctic domain further, we used the D values from non-parametric K–S tests as a measure of the maximum offset between the density distributions (Fig. 1a). D values represent the maximum vertical distance between the cumulative distribution function described by the INTERACT network (with or without Russia) and the cumulative distribution function describing the pan-Arctic domain. The null hypothesis is that both groups were sampled from identical distributions, and significant K–S tests thus indicate that distributions differ. As a yardstick for the magnitude of the potential bias, we used the D values derived from comparing the projected shifts in ecosystem conditions between the years 2016–2020 and 2096–2100 (see above). To visualize potential biases further, we extracted the first, second (median) and third quartiles (Q1–Q3) from the density distributions, as general indicators of the ecosystem conditions at the INTERACT stations (with and without Russian stations) and across the pan-Arctic region (Fig. 1b).
To visualize the impacts of the exclusion of Russia from INTERACT as loss of ecoregion representation across the pan-Arctic region, we calculated the distribution of INTERACT stations per ecoregion with and without Russian stations. The ecoregions in Fig. 2 were defined as follows: (1) the High Arctic region covered the bioclimatic subzones A, B and C, from the Circumpolar Arctic Vegetation Map30 (CAVM; accessible in ref. 31), (2) the Low Arctic region covered the CAVM subzones D and E and (3) the Sub-Arctic region is derived from the tundra forest subzone in the Ecoregion 2017 classification32 (available at https://ecoregions.appspot.com/) situated below the tree line. The Boreal region corresponded to the Ecoregion 2017 boreal forest subzone, and the Alpine region covered altitudes above 1,000 m but below the tree line. The latter was derived by the ArcticDEM product33 (accessible in ref. 34).
Data and analysis caveats
Incorporating in situ field information holds the potential to reduce the anticipated uncertainties associated with the type of analysis presented in this paper. A growing abundance of high-temporal, quality-checked, long-term data is now accessible through online repositories for both scientific papers and data (for example, thematic scientific networks like FLUXNET, International Permafrost Association and so on). However, a substantial gap still remains in terms of a unified, coordinated approach to harmonize and integrate diverse monitoring data from various sources (spanning across countries or disciplines), as highlighted in refs. 8,9. Moreover, the absence of standardized methodologies (such as instrument branding, variable units or temporal resolutions) among research stations presents a challenge to comprehensive in situ field data intercomparisons.Additionally, while robust spatial products are available, such as re-analysis climate forcing (for example, ERA5 (ref. 35)), remote sensing products (for example, ESA Climate Change Initiative for vegetation-related variables such as biomass36) and machine learning-derived estimates (for example, FLUXCOM for terrestrial C fluxes37), it is important to acknowledge that such datasets are associated with inherent biases and uncertainties (as highlighted in, for example, ref. 38). Similarly, bottom-up exercises from land cover/vegetation type classification maps, though valuable for upscaling, can be affected by heterogeneity issues and uncertainties, leading to potential biases when extrapolating from such analyses.
Coupled climate models remain the best and currently the only tools available for evaluating shifts and trends in the future climate system13, along with the associated ecosystem responses and feedback loops39. While large-scale climate models provide credible and convincing numerical estimations for recent past and future scenarios on a regional-to-global scale40, differences in model performance are far from perfection41. For instance, model uncertainties stem from various sources, including differences in model structure and parameterization (for example, ref. 42), external forcing (for example, ref. 43) and emission scenarios (for example, ref. 44). Such limitations introduce uncertainties on both atmospheric (for example, refs. 45,46) and ecosystem processes (for example, refs. 47,48), particularly those related to land (for example, refs. 38,49). Currently, the terrestrial carbon cycle remains the least constrained component of the global carbon budget (for example, ref. 50). For example, the models account for equilibrium states, but it has been recognized since the 1980s that plant species are unlikely to relocate as fast as their appropriate climate envelopes (for example, ref. 51). Also, models of treeline movement overestimate latitudinal relocation by up to 2,000 times52. A consequence of this is that some vegetation will remain in climate envelopes to which they are not adapted and will/are experiencing impacts of extreme events. These impacts have local implications53, and some have regional impacts, for example, the movement of the Circum-Arctic treeline54 and the impacts of thawing permafrost on wetland dynamics and vegetation/biodiversity6,55.
Data availability
All CMIP6 modelling datasets used in this study can be accessed and downloaded freely from ESGF repositories (for example, https://esgf-node.llnl.gov/projects/cmip6/ and https://esgf-data.dkrz.de/search/cmip6-dkrz/). Locations of Arctic research stations are available at the INTERACT GIS portal https://www.interact-gis.org/Home/Stations. The source datasets generated and/or analysed during the current study are provided, corresponding to each figure and table. Any additional data are available from the corresponding author. Source data are provided with this paper.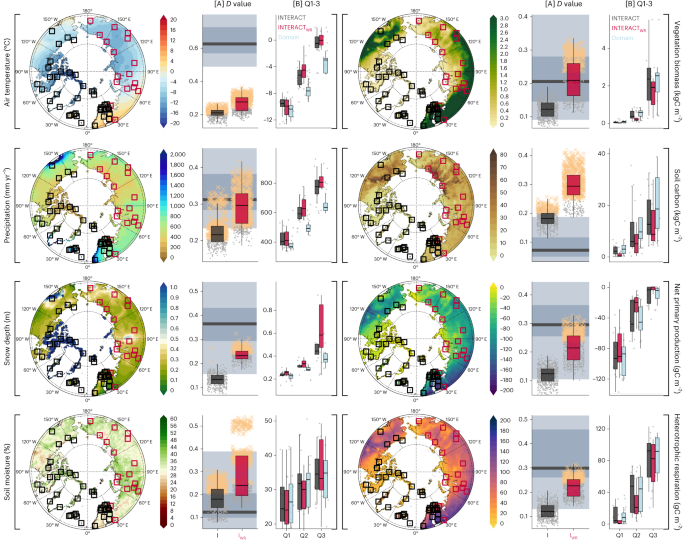
Towards an increasingly biased view on Arctic change - Nature Climate Change
The authors investigate the impacts of excluding ecosystem data from Russian stations in the Arctic. While the current network of Arctic stations is already biased, the exclusion of Russian stations lowers representativeness and creates further biases that can rival end-of-century climate change...
